Fluoroscopic Imaging Automation through Linear Regression and Diagnosing through Convolutional Neural Networks
DOI:
https://doi.org/10.5530/ctbp.2023.3s.62Keywords:
Fluoroscopic Imaging, Dosage Area Product (DAP), Kerma Area Product (KAP), Automated Scope, Linear Regression, Convolutional Neural Networks (CNN)Abstract
Fluoroscopic imaging is a medical and pharmaceutical biotechnology imaging process that uses x-rays to capture moving pictures of the internal parts and organs of the body. These images can aid in the detection of fractures and other types of bodily injury. Dosage Area Product (DAP) (also known as Kerma Area Product (KAP)) is an important statistic used to quantify the level of radiation dose for each organ. It is determined by multiplying the absorbed dosage by the area of the x-ray field. The standard approach for determining the primary components in fluoroscopic pictures is challenging due to the high possibility of error rates. This paper proposes an automated approach for fluoroscopic imaging using Linear Regression and Convolutional Neural Networks (CNN). This approach aims to improve the accuracy and reliability of identifying primary components in fluoroscopic images.
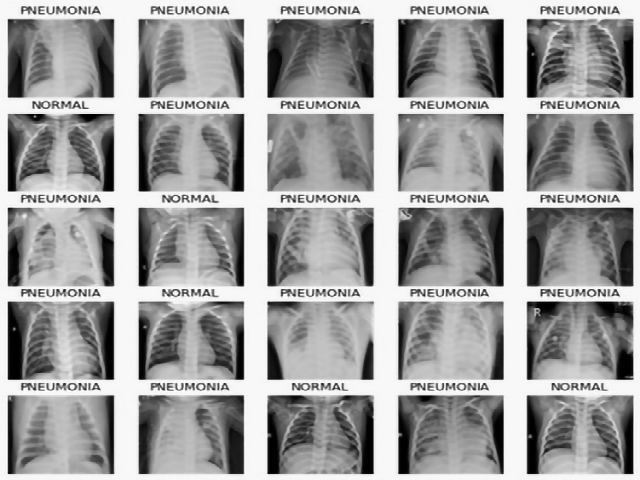